NextCODE Brings the deCODE Data Architecture to Tumor Analysis
By Bio-IT World Staff
June 12, 2014 | Last March, when Bio-IT World’s founding editor interviewed Kari Stefansson of deCODE genetics, the fate of a program called Clinical Sequence Miner was up in the air. The software platform was a promising tool for seeking out disease-causing genetic variants, either in specific patients, or for research studies in a clinical setting. Sequence Miner had some appealing features: it was a self-contained pipeline, using raw sequence data as an input instead of sitting on top of several layers of informatics, and its interface was built for clinicians who may have minimal experience with genetic analysis tools.
But deCODE had just been acquired by Amgen, and the large pharmaceutical company was more interested in deCODE’s skills as a basic research organization, dredging up leads on drug targets from its massive database of Icelandic genomes.
“Amgen took over deCODE mainly to make sure that Kari would be unfettered to continue making discoveries in the Icelandic population,” says Jeff Gulcher, the co-founder of deCODE with Stefansson who was then serving as the company’s Chief Scientific Officer. “They didn’t want to get involved with diagnostics.”
Amgen did end up jettisoning Sequence Miner, but it didn’t bury the platform. Instead, the Sequence Miner technology has become the core of a new business, NextCODE Health, and continues to spin out new applications — including, most recently, a tool for clinical cancer research that analyzes tumors on the level of whole genomes.
Intelligent Architecture
Gulcher became President and CSO of NextCODE when the new company officially separated from deCODE in October 2013. (See, “NextCODE Health Launches deCODE’s Clinical Genomics Platform.”) Although direct cooperation between the two companies is minimal, Gulcher explains that the experience of working with deCODE’s huge database of whole genomes* is foundational to his own company’s services. That’s because deCODE was one of the earliest organizations to struggle with truly large genetic datasets, tracking millions of variants across thousands of individuals.
“We invented a new database infrastructure, a way of organizing sequence or genetic data, way back about ten years ago when we were in the DNA chip era,” Gulcher tells Bio-IT World. “We were measuring about a million SNP markers per patient [at the time]… Once we got up to about five or ten thousand patients, it was very hard to get the data out quickly. You had an enormous input-output problem when it came to extracting the data in order to feed a statistical algorithm.”
Jeff Gulcher, President and CSO of NextCODE Health. Image credit: NextCODE
The solution deCODE devised was the GOR (Genomic Ordered Relations) database. GOR understands the genome in terms of chromosomes, where each genetic variant occupies a physical position, rather than as a continuous string of sequence. When searching for a variant, tools in the GOR architecture don’t have to scan all the sequence data on each individual; they retrieve the variant straight from its location.
GOR also stands out for keeping every stage of genetic analysis in-house, from organizing the raw sequencing reads, to annotating variants with information about their effects on protein formation, function, and potentially on health. In annotation, NextCODE can draw on a set of 40 million variants that deCODE has found in the Icelandic population, which are linked to the clinical histories of individual Icelanders. “We keep all the raw sequence and variant data separate from all the annotation data,” adds Gulcher. “What that means is the annotation files can be updated frequently, without rewriting all the raw data in the database,” making it easy to keep GOR’s knowledge up-to-date as both public databases and deCODE amass more information.
This flexibility has helped NextCODE quickly release offshoots of Sequence Miner, which rests on top of the GOR database. NextCODE’s flagship product is Clinical Sequence Analyzer (CSA), which is used to make clinical diagnoses, usually of pediatric patients with rare hereditary diseases. CSA draws on a large knowledge base, but its appeal rests equally on its speed and ease of use. Thanks to the GOR infrastructure’s ability to rapidly cycle between personal sequence data and broad clinical knowledge, clinicians can simply type in a patient’s symptoms, and CSA will search the patient’s whole genome for variants that may be relevant. That platform, along with the more research-oriented Sequence Miner, has been placed most prominently in the Molecular Core at Boston Children’s Hospital, where it serves a large collection of Boston-area care centers.
Targeting Cancer
Last week, however, NextCODE representatives were demonstrating a different platform at the annual meeting of the American Society of Clinical Oncology in Chicago. The Tumor Mutation Analyzer (TMA), the latest addition to NextCODE’s suite, turns the GOR architecture to one of the most daunting big data problems geneticists face when working with single patients: cancer genetics.
TMA takes whole exome or whole genome sequence from a patient’s tumor cells, as well as normal cells, and isolates the variants likely to be cancer drivers. Gulcher compares it to the panels used by Foundation Medicine, a leading tumor analysis company. “What Foundation Medicine does is sequence a limited number of genes — about 200, but it’s the 200 known genes that are more likely to have been seen before, and have some drugs developed against those particular pathways,” he says. “It’s a valuable product… [but] we think the future is looking at everything, not just 200 genes.”
TMA uses two popular algorithms to flag potential cancer-related variants: the open source VarScan 2, and MuTect, which NextCODE licenses from Appistry and the Broad Institute. Users can further narrow the variants called to those that have the most dramatic effect on protein function, or sort them by molecular pathway or associated drugs.
The distinguishing feature of TMA, though, is the sheer depth of the data it stores. Like in CSA or Sequence Miner, users can always trace a variant back to a visual map of the genome with the raw reads aligned, to verify that the variant is real and correctly described. The genome browser view can also highlight important context for a variant’s impact — for instance, showing how much of a protein is mistranslated due to a truncating mutation. In TMA, such complete information is retained on the raw data that users can check the sequencing coverage of every individual base.
“We have three billion columns to keep track of” in that coverage database, says Gulcher. “Who’s crazy enough to store three billion columns, right? Well, we do it, because it allows you to have a better handle on copy number variation, or look for de novo mutations much more easily, because you know the quality of the sequencing… The GOR database opens up a new world. It allows you to use as much data as exists. You don’t have to skimp on the data, you don’t have to lose the data to compress.”
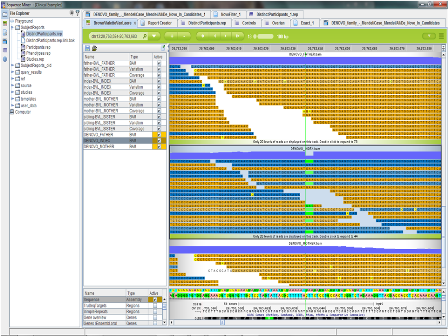
This level of detail is especially important in cancer genetics, where the chances of finding previously unknown variants are very high, and even if a mutation is successfully targeted with a course of treatment, another potential driver is often waiting in the wings. With no practical limit on how much data can be placed in the GOR database for a specific case, TMA has also been used in studies that add RNAseq data to capture the expression-level effects of mutations, or reevaluate whole genome sequences taken from a tumor before and after treatment to see what new mutations gained ground.
At present, TMA is meant for clinical research only; while it does draw connections between variants and treatment options, using that information in patient care demands a robust regulatory environment. “Our goal is to work with medical centers initially, to use this information for their own CLIA laboratories,” says Gulcher. In the long term, however, the goal is to turn TMA into a user-friendly clinical tool like CSA, likely with some standard analysis pipelines. These are already a prominent feature of CSA, where users can ask the platform to show them all the variants related to cardiomyopathy, or all those on the list of incidental findings that the American College of Medical Genetics recommends reporting to patients.
“It’s a one-step thing, and it gives you a nice summary report along with some detail, and you can stop there if you want,” says Gulcher. If TMA too is adapted for clinical use, these kinds of reports will be a useful output for the treating physician who ultimately has to make the calls on introducing new therapies. Meanwhile, specialists with more experience in genetics would be free to dig as deeply into the data as they like. “You do have specialty physicians who are involved with tumor analysis today, so we see those as the sort of physicians who ultimately could make use of the Tumor Mutation Analyzer,” Gulcher adds.
In the meantime, NextCODE is opening up TMA to research partners generating genome-scale data on cancer cases. Like CSA or Sequence Miner, TMA can be hosted remotely at deCODE’s facilities in Iceland, or a private iteration of the GOR database can be set up on local servers or cloud services.
*DeCODE boasts whole genome information on 350,000 individuals, though this figure is somewhat misleading. The company has directly sequenced the whole genomes of around 4,000 Icelanders, which is itself a significant number. But Iceland keeps detailed health and genealogy records on its highly genetically isolated population, and deCODE uses that information to infer the genetic variants carried by hundreds of thousands of others. On a case-by-case basis these hypothetical genomes are far from perfectly reliable — you certainly wouldn’t want to use them for a clinical diagnosis — but statistically over the whole population they are very useful.
It should also be noted that deCODE’s efforts to sequence more and more of the population of Iceland, with encouragement from the Icelandic government, have not been without controversy. If you’re interested, you can read a critical take on the programs, by a popular Icelandic political observer, here. What is not in doubt is the research value of the database, which has made deCODE a publication powerhouse, particularly in the areas of rare heritable diseases, and small genetic contributors to common chronic disease.